Einführung durch der Professorin
Prof. Dr. Xiaoying Zhuang
Professor für Mechanik von für Multiskalen- und Multiphysik-Probleme
Im Jahr 2014 kam ich mit einem Marie Curie International Incoming Fellowship der Europäischen Union (damals im Rahmen des FP7-Programms) nach Deutschland. Das Fellowship endete, als ich im Dezember 2015 mein Sofja-Kovalevskaja-Programm dank der Alexander von Humboldt-Stiftung begann. Mein Sofja-Programm wurde am Institut für Kontinuumsmechanik (IKM) durchgeführt, und ich hatte das Glück, mit Prof. Peter Wriggers zusammenzuarbeiten. Dank meiner früheren Kollegen am IKM konnte ich mich gut entwickeln und genoss die Zusammenarbeit. Im Jahr 2018 wurde ich mit dem Leibnitz-Preis und dem ERC Starting Grant des Horizon 2020-Programms der Europäischen Union ausgezeichnet. 2019 erhielt ich das Heisenberg-Professorenprogramm und... Ich begann das Programm Anfang 2020... ja, genau fast zur gleichen Zeit wie der Beginn der Pandemie. Wie Sie sich vorstellen können, war der Aufbau einer unabhängigen Forschungsgruppe im Jahr 2020 eine schwierige, aber interessante und lohnende Erfahrung, die ich in Erinnerung behalten werde.
Mein Hauptforschungsbereich liegt in der Computational Mechanics and Machine Learning zur Lösung von partiellen Differentialgleichungen in Ingenieurwissenschaften und Naturwissenschaften, insbesondere für multiskalige und multiphysikalische Probleme. Ich habe Berechnungsmethoden für die folgenden Themen entwickelt:
In den vergangenen Jahren gab es viele interessante Erkenntnisse und Fortschritte. Im Folgenden werde ich einige dieser Ergebnisse hervorheben und teilen.
Lehre
-
Scientific machine learning (SciML)
In this course, the students will be introduced to the foundamental concepts, theories, computations as well as applications of machine learning. It will start with the foundemantal introduction of machine learning algorithms oriented to different learning tasks. After the students have acquired a basic knowledge of machine learning, we will further proceed to scientific machine learning. Detailed examples involved in various scenes of scientific machine learning applications covering applications will be expounded for students from different backgrounds. Current availabe software that can be used for scientific machine learning and their limiations will be shown and discussed.
Graduates at the end of this course should be familiar with those classical machine learning models and qualified to setup their own machine learning model with those open-source python libraries. At the end of the course, the students are expected to be able to utilize the prior knowledge in their own research filed in building the SciML models and understand the validity and limits of their results. They shall be experienced on understanding and discussing the state of the art literature in the scientific machine learning and on the defense of their findings by an oral presentation of a selected problem. -
Fracture of Materials and Fracture Mechanics
TBA.
-
Introduction to Multiscale and Multiphysics Modelling
TBA.
FINANZIERUNG
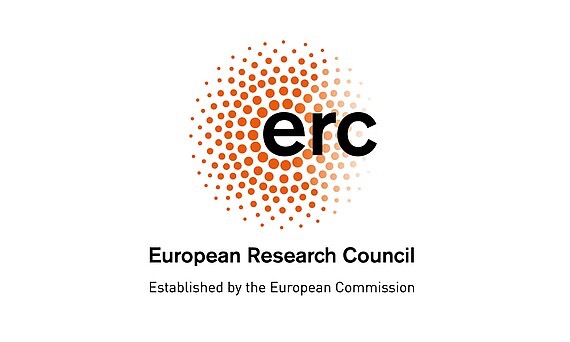
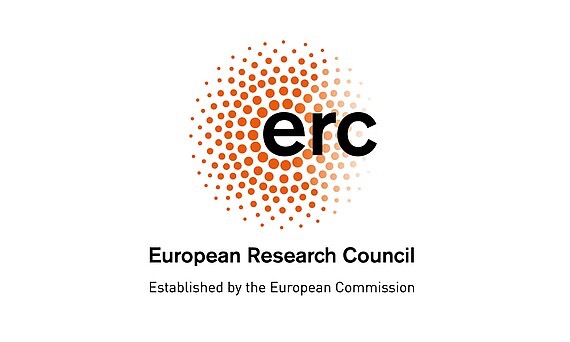
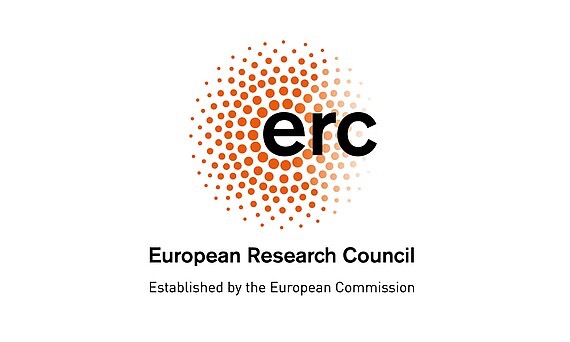
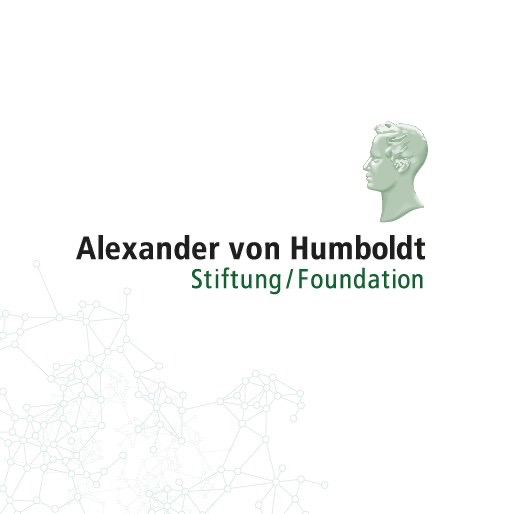
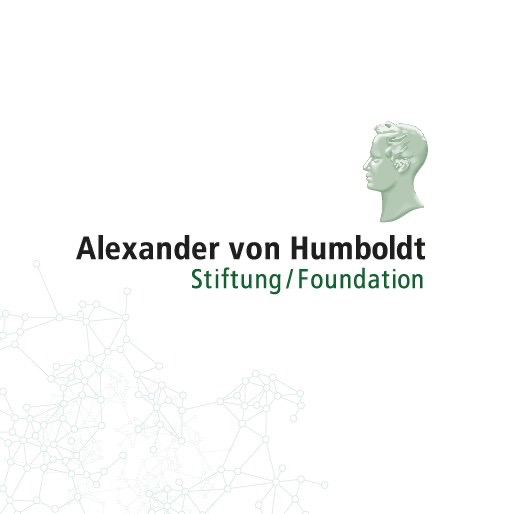
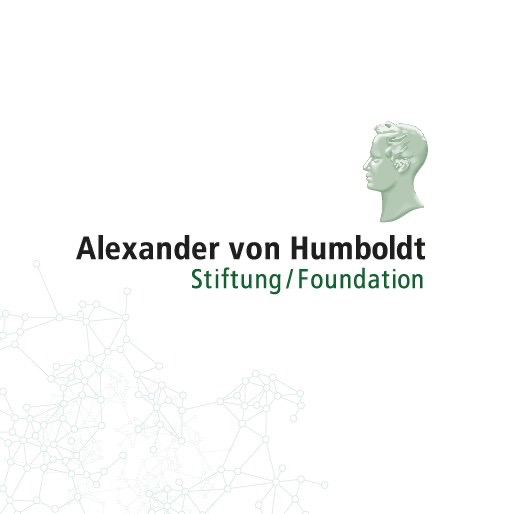
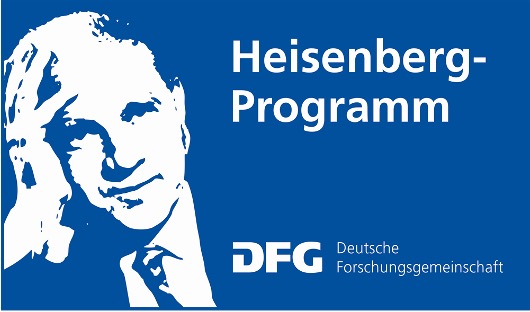
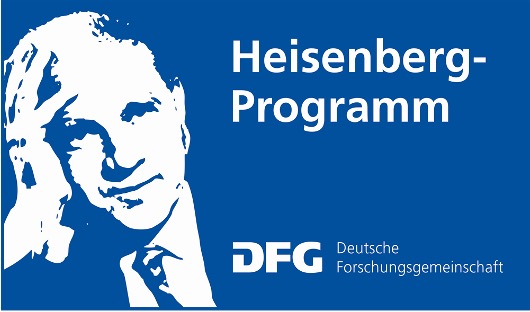
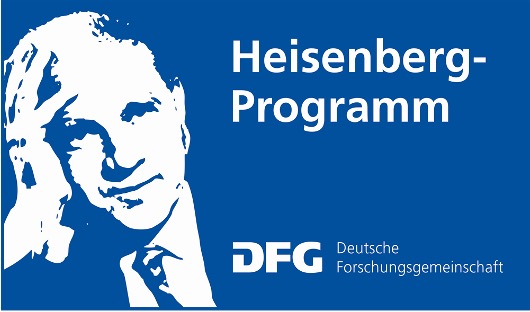
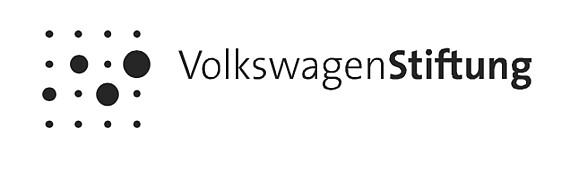
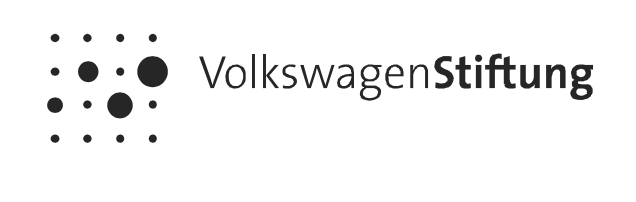
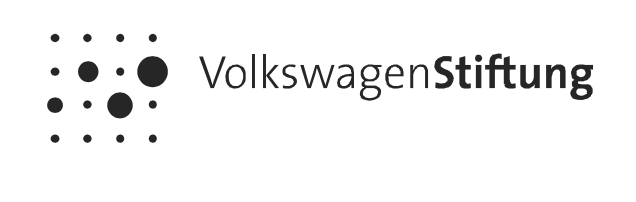